I'm no longer browsing the web; I'm consuming AI answers instead.
I love and adore today's so-called AI answer engines: tools like ChatGPT, Perplexity, Grok, Copilot, and Gemini. I use them at least a dozen times throughout the day, and I'm sure you use them frequently as well. Their convenience is undeniable.
I turn to these tools to save me some clicks, but I give up control for that convenience. What I gain in efficiency—no longer needing to browse through slow-loading ad-heavy websites just to find out how to tie a Windsor knot or grab a recipe—I lose in the personality, idiosyncrasy, and creativity that makes the web feel authentic. We've come to expect, seek, and rely on this.
Browsing with traditional search engines wasn't exactly seamless but you were in the driver's seat. You would occasionally end up on some obscure personal site or forum that was unexpectedly right up your alley.
Those magical detours defined our web experience, enriching us with insight and creativity while establishing human connections. Today's instant answers with these AI tools sacrifice this beautiful chaos that once made the internet so captivating. But there's hope—a new kind of browsing experience might just be around the corner.
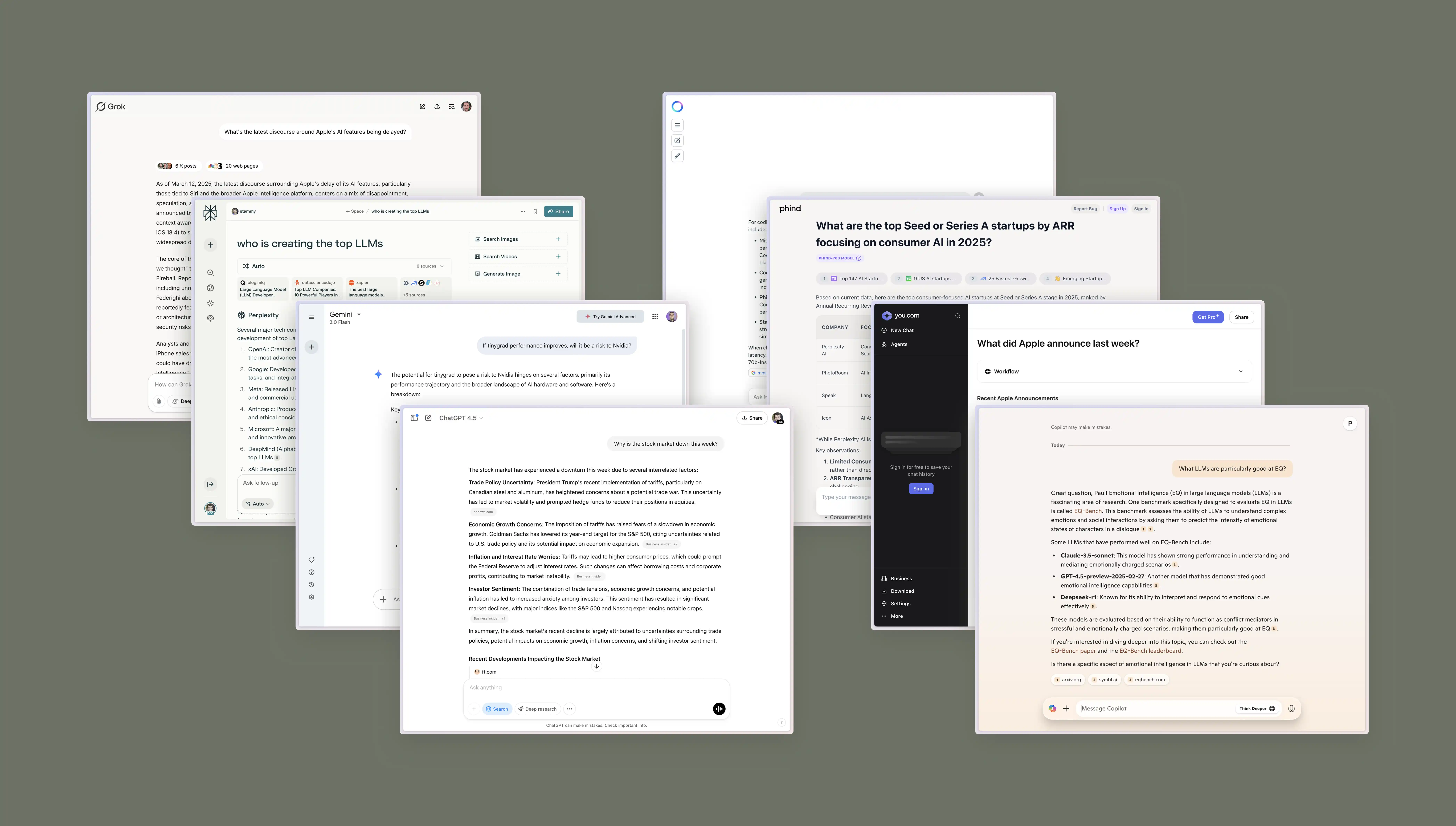
We're increasingly experiencing the web like this.
Before we had AI answer engines, and before we had search engines we just had lists of links with web directories like aliweb, Yahoo! Directory and dmoz. You'd tediously wade through these directories to find and absorb content you were interested in, or just to explore and tinker. Everything online was created by people and you were getting a glimpse into their world with each site.
The web grew. We gained search engines, blogs, feed readers, social media and more. While there were new ways of creating content and new ways of consuming, when you really needed something you'd still turn to a search engine and click around until you found what you needed.
This led to inevitable moments of delightful and serendipitous discovery. There was real joy in discovering another unique voice online, someone whose articles and interests were right up your alley. Their style of writing lended itself to being devoured in one sitting, while you scan their site to see how you can bookmark or subscribe to keep tabs on their latest works.
It wasn't just about stumbling upon a random personal blog that was a fun occasion. It was finding communities you didn't know existed. Even just recently as I've been upgrading my iMac G3 and running into some issues, I came across a forum for Mac OS 9 enthusiasts that was chockfull of the exact type of content I was looking for. It was not easy finding it. It didn't happen in one sitting, or with one tool and ended up being something I caught in passing that someone said in a video on a blog. But the payoff was worth it for that moment.
Serendipity while browsing the web wasn't just a byproduct of how the web began to form. It stretched our empathy by exposing us to diverse voices, nudged us out of echo chambers, and kept our web from becoming monotonous.
That style of surfing the web is fading away. What social media hasn't already taken over, or search engines haven't already diluted by sending us to ad-laden mainstream sites, is now steadily being eroded by AI-powered answer engines.
AI answer engines are phenomenal in what they do. I can fire off a prompt and relatively quickly get a blurb with the exact information I need. It really can't be overstated how great they are.
But I've lost something profound in the process—the joy of the unexpected detour. I'm not really browsing the web anymore. At best, I'm just browsing AI interpretations of a limited slice of the web. A slice that I didn't even pick or have control over. My surface area for discovery is now whatever these tools decide to show me.
It's not just me. Traditional search engines are evolving into answer engines. Zero-click searches are rising and will continue to rise. Nearly 60% of Google searches now end without the user clicking to any site. And now Google is experimenting with "AI mode"1 to take this to the next level.
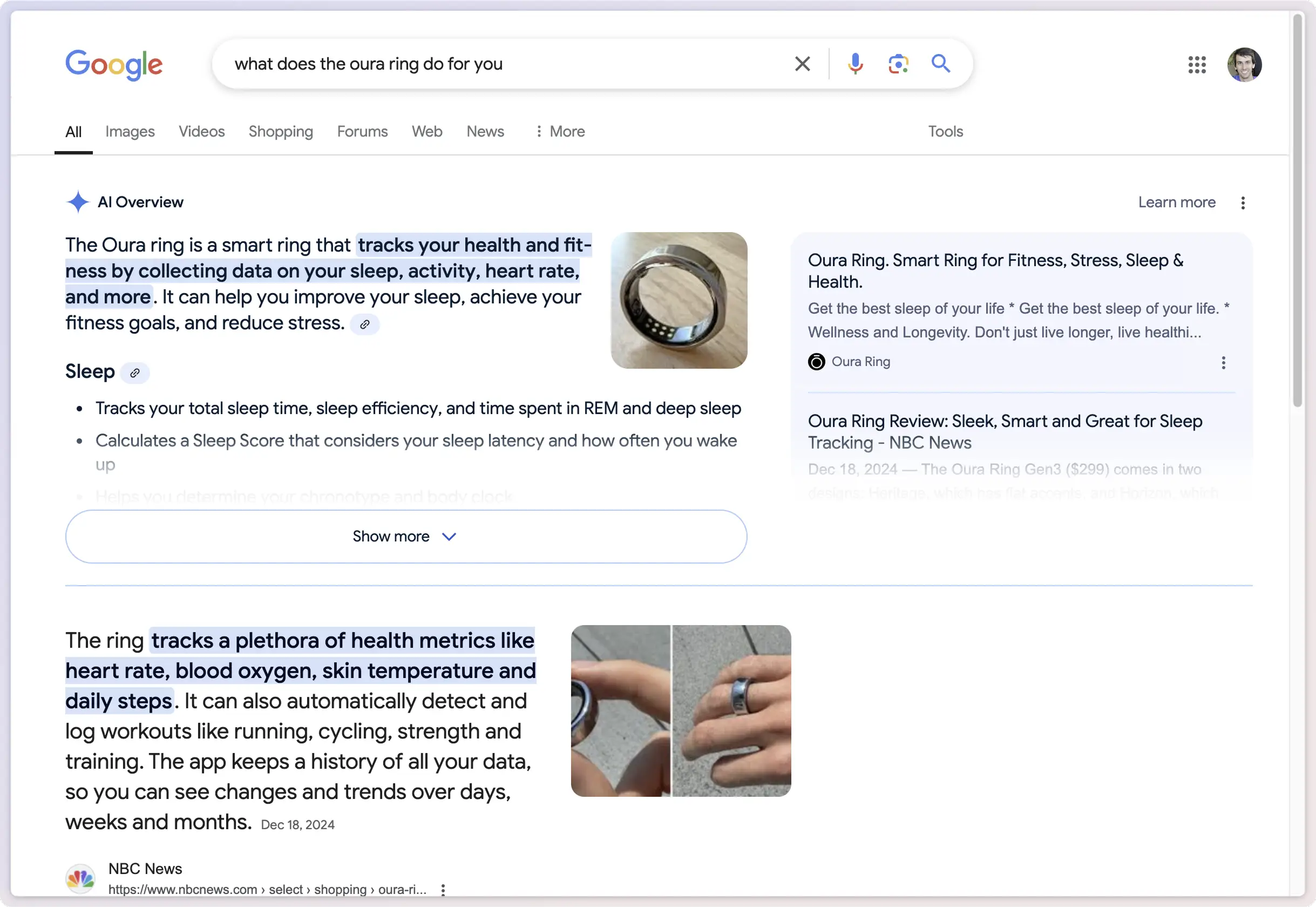
Traditional search engines are becoming answer engines. Google's prominent Search Generative Experience (SGE) and it's focal CTA to show more. I love my Oura ring for what it's worth.
Core issues
Is this really a problem?
This is just another classic example of technology evolving. Who cares if it's a bit harder to find some smaller websites? I see a few issues at play here, and it extends beyond just web browsing. At a high level, these AI answer engines lack transparency in how they work, remove control, and homogenize what's left, sucking all vibrancy out of what makes the web special.
-
1) Deprioritized attribution
These tools largely show sources as a tiny footnote link. As an industry we started using that design pattern to link to receipts to prove the LLM was not hallucinating. We need to highlight great content when it's most relevant, not bury it with a link and a little generic hovercard or popover.
-
2) The black box of search
How do these tools index and rank the web? How do they decide what content is most relevant to a given question or user? They largely prioritize mainstream sources. How do they interpret your question and turn it into keywords to search their bespoke web index? How do they know when they've retrieved enough info? We lost the agency over these things we had with search engines.
-
3) Summary homogenization and mode collapse
Even with access to diverse web sources, responses from these tools can feel a bit... off. Fine for when you're only looking to fetch basic facts, but limiting and less vibrant for anything else. It's like having access to every book in the world, but only being able to read them summarized by the same person with the same writing style.
1) Deprioritized attribution
The way these AI answer engines approach attribution leaves a lot to be desired. The early days of LLMs were especially riddled with hallucinations. The industry began using tiny numeric footnote citations to show that they had the receipts and there was actual data behind each sentence. I even did the same (albeit showing screenshot thumbnails too) when designing and building this feature.
In addition, these tools try to impress upon us that they are mighty and have referenced some huge number of webpages while coming up with each response. They display this information as a pile of favicons or list of sources, with no easy way to understand why they were included or how they were used. You're not meant to wade through it, nor should you have to.
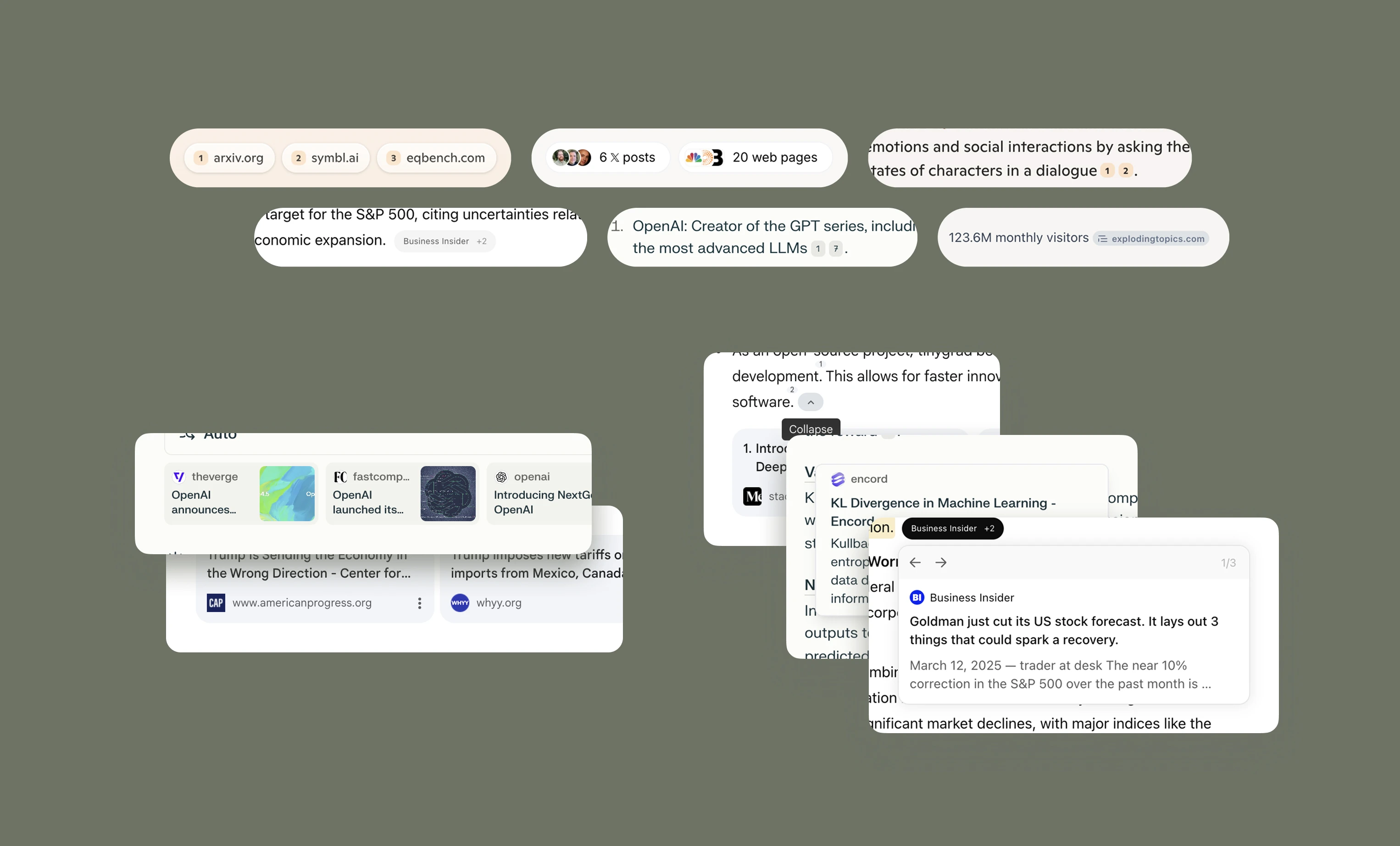
Web source attribution shown on these tools with inline citations, source bars, and hover cards.
Each source receives roughly the same visual representation with these tools. There's no transparency if it's from a reputable mainstream news site, a single author that's a domain expert given their past works, or a site you've frequented before. At most you'll get an expanded card or sidebar showing more the favicon, domain name, article title along with truncated content.
Some tools do it better than others, including the domain name as the citation instead of a number, or listing a few sources in the footnotes. But that still doesn't tell me much about the site, author, why this particular source was selected, and how its content was used. There's lots of signals that can be used to help guide understanding here.
It would also be interesting to also consider LLMs employing a system similar to X's Community Notes (née Birdwatch when I worked at Twitter) to clarify the context of and add perspective of web sources. For example, a source might seem upstanding but then you discover a whole Hacker News thread explaining in detail why it's not accurate.2
And finally, if you take a particularly dystopian view it's not hard to see potential second order effects of these tools with their deprioritized attribution. Perhaps authors will begin focusing on their own empires, where they have control over how their content is seen and monetized—such as moving it to private newsletters, away from the prying eyes of LLMs. AI companies may license data from bigger sites that do this, but definitely not your personal blog or sites on the small web.
Better or more prominent attribution isn't the solution to everything. It's a way of framing content to reflecting a broader range of perspectives, and elevating enough signals to allow the reader to understand what they're presented and why. Simply sampling the top X keyword results on a topic isn't enough to do this or reduce the echo chamber feeling; you also need greater control, understanding, and transparency in the search process.
2) The AI search black box
We also face lack of transparency regarding how these tools search the web to gather and process information needed to answer your questions. There is the potential for search and ranking bias, or lack of clarity at the least. Questions like "How are they creating their search indexes?", "What do they value when ranking sites?", and "How do they select a set of web results for user queries?" get elevated.
AI answer engines make countless hidden decisions about what sources matter and what information is relevant—all without your input or awareness. You get the illusion of comprehensiveness, when in reality you're getting a narrow slice of available information filtered for you.
These tools each have their own way of searching the web. Some may lean on third-party search indexes like Bing (ChatGPT) or X/Brave Search (Grok) but conduct their own crawling and ranking, while others handle everything independently. You've probably become fairly accustomed to how results feel in Google or DuckDuckGo, and you have a good grasp of how to wrangle them to find what you're looking for.
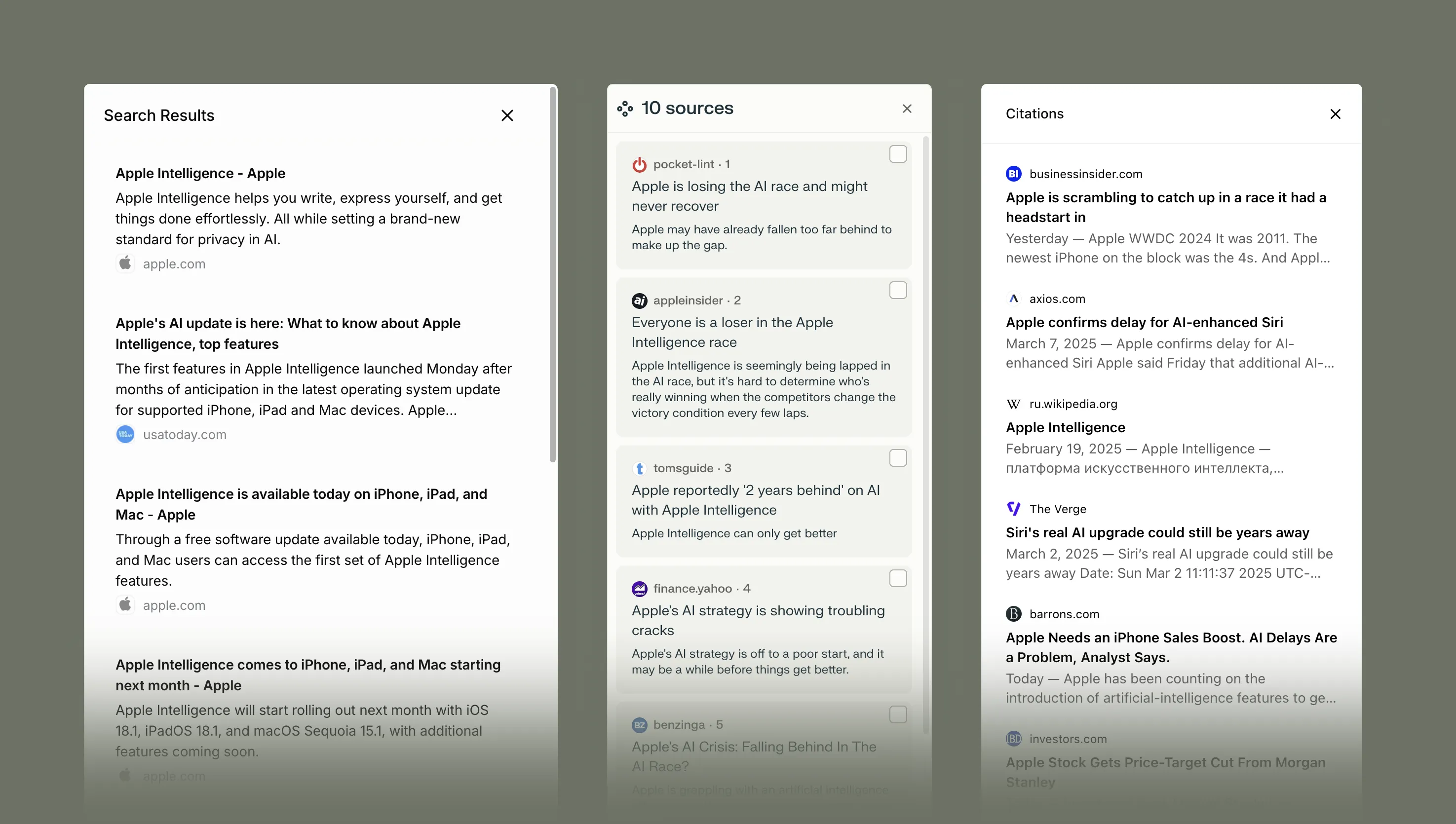
Expanded web sources shown in Grok, Perplexity, and ChatGPT. All lack context about what kinds of sites these are, why they were selected, and how they were used.
Now we're dealing with a new system entirely, but this time you're disarmed—you've lost your agency to fiddle with keywords, endlessly navigate pages, decide when to try a new query because one wasn't fruitful, and so on. Of course, you can always ask the AI answer engines a different question or provide a follow-up, but you still lack control over how they're translating your prompt into an index search.
Perplexity, for example, tries to provide greater transparency when doing a Pro search by showing the exact keywords they used to search their web index. However, I find this approach gives me even less confidence in what it was doing. Customers approach these tools thinking they are all-knowing AI supercomputers... and then see their thoughtfully-posed question get dumbed down to a 4 word search? I have no control over that process nor when it decides to keep searching.
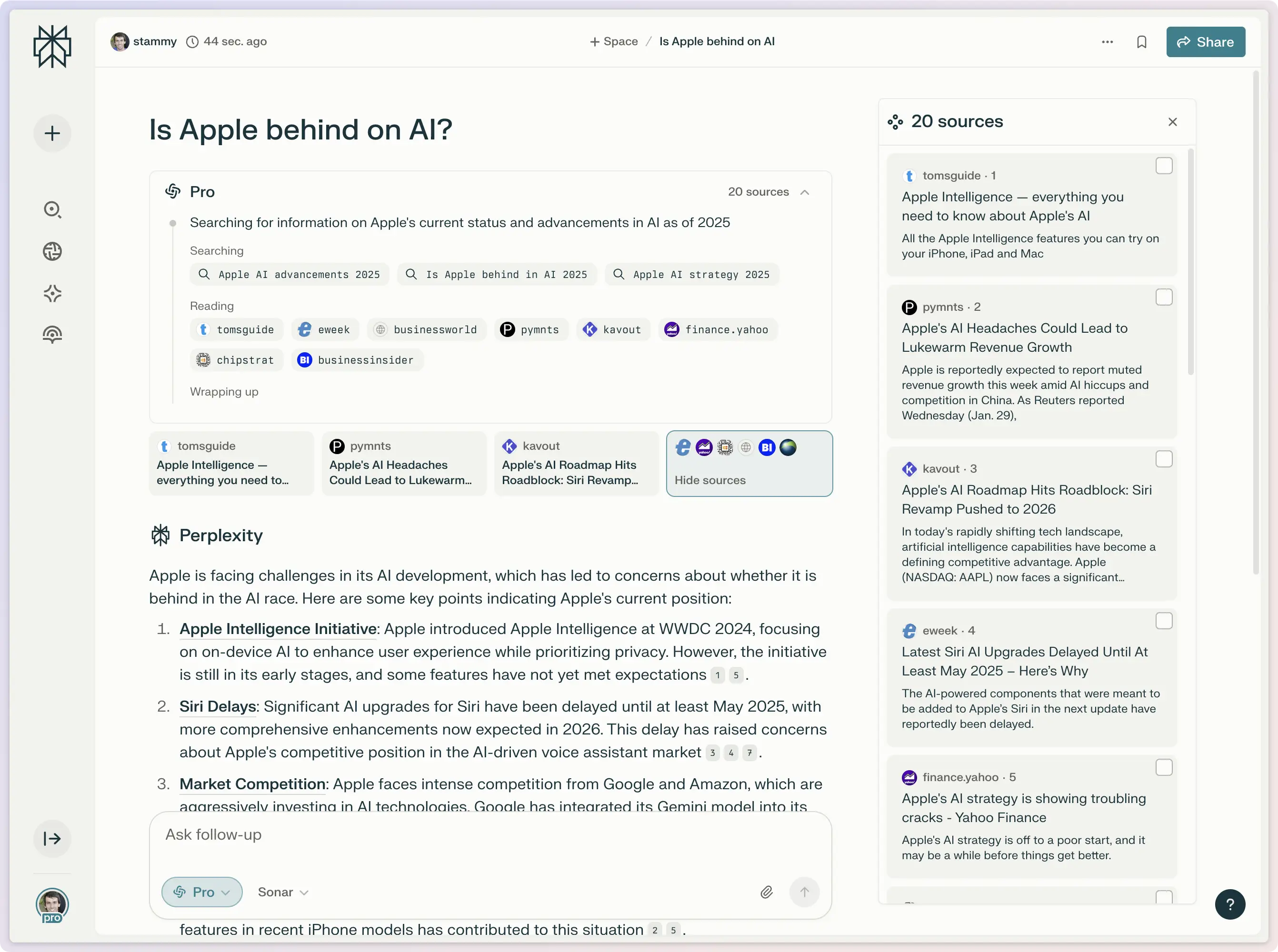
Perplexity Pro search showing different keywords used for a web search based on the question.
This screenshot also perfectly illustrates the core problem. The entire Internet has been talking about Gruber's post about Apple Intelligence, that should have been included as a source but I have no clarity into how it ranked, searched, and selected these mostly mainstream sources.
What that type of UI does accomplish well is the job of communicating progress successfully during a long-running task. Even more intricate UIs exist, such as those from ChatGPT, Perplexity, Gemini, and Grok for deep research3 tasks that providing a peek into CoT reasoning. ChatGPT's deep research does do one novel thing when presenting sources though, it shows a tiny snippet of how that result was used.
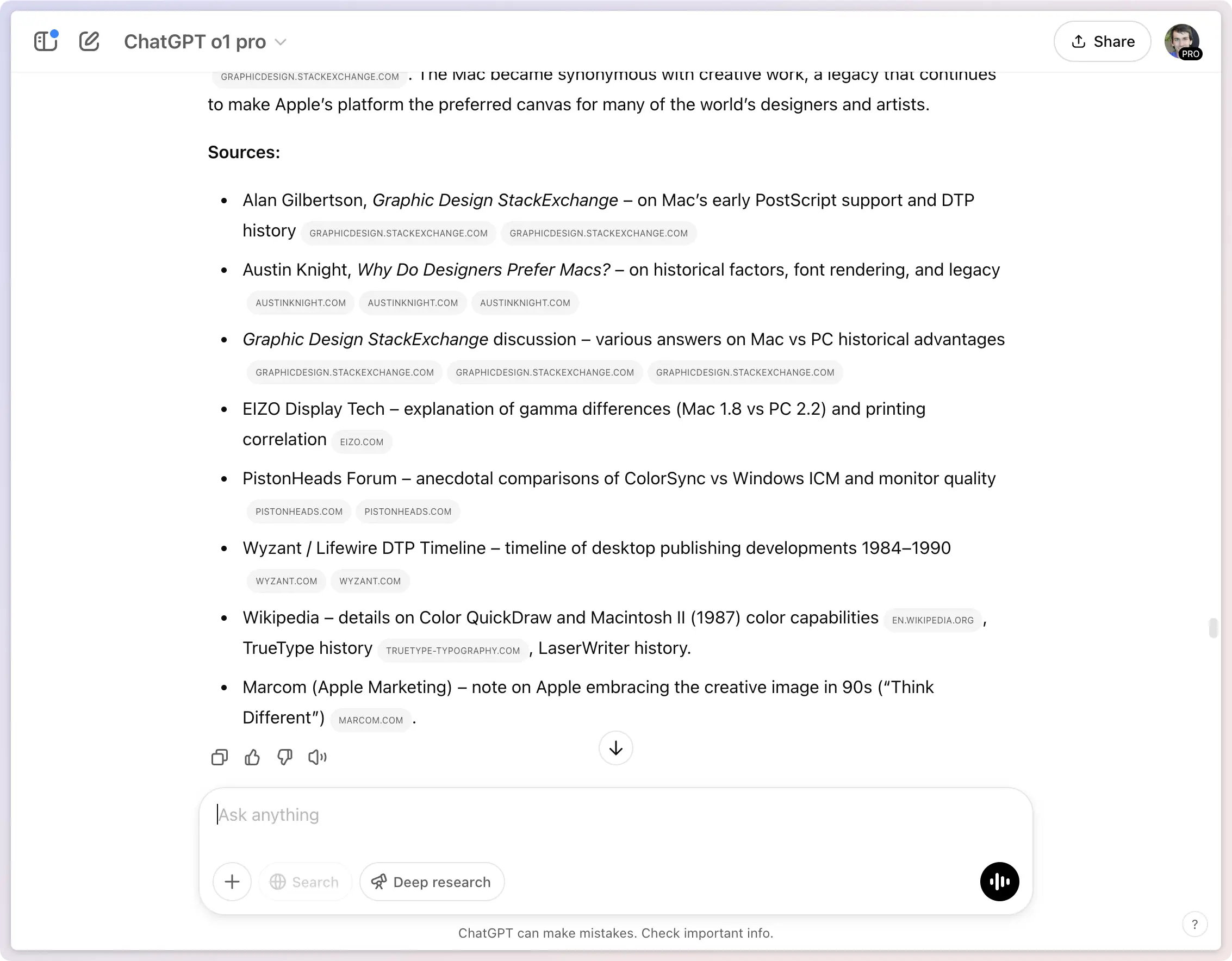
ChatGPT's deep research starts to add a bit of context about what a source was used for: a welcome step in the right direction.
There's also risk on the other side of it: letting users assume that the response they get is the truth. Maybe the cursory web search the answer engine did behind the scenes wasn't thorough enough? This is especially the case when dealing with niche topics. (Grok does the absolute worst here: for niche topics it won't reference any websites at all!) You've really got to scour the web to find what you need. If these tools are just a few keyword searches in their search index, that won't cut it. They don't know when to keep searching or ask for further clarification.
I realize that having to fiddle with search engines, while it can be seen as a control, can also be a nuisance at times. I'm not trying to be too dismissive here.
The huge benefit I'm flying past is that LLMs do a pretty solid job of understanding your intent and nuance—a crucial trait, given that most people still struggle to write great questions. Though I also think we should do more to guide people here; Elicit does a nice job with this UI to suggest different ways to ask precise questions.
Research tool Elicit helps its users determine if their question is strong enough and suggests ways to improve.
We deserve so much more here. If AI tools understand our intent so well, they should deliver much more natural, interesting, and diverse results that feel indistinguishable from having a panel of experts at your side.
3) Summary homogenization and mode collapse
You know the feeling. AI-generated content often doesn't feel great. It feels slightly off; vaguely mechanical, even predictable. LLMs are largely consensus machines. In the pursuit of zero bias, you end up systematically smoothing out any novel perspectives or viewpoints introduced through these already not-too-diverse web sources used as context.
This feeling where models lose their creativity after post-training is often called mode collapse. Its makes LLMs particularly unsuitable for tasks like creative writing. Sudowrite, an AI writing tool, specifically addressed this problem of "AI-isms" when announcing their new writing model. And just recently, OpenAI mentioned they're working on a model made for creative writing.4
When you try to please everyone, you wow no one.
The way we build and train LLMs has led us to this. I could keep going down the rabbit hole and discuss the need for LLMs to get better at creativity. This is another thing you feel, even if you can't quite put your finger on it.
People could tell when Sonnet 3.7 felt a bit worse than 3.5. They were impressed when GPT 4.5 could do more despite not being a benchmark-killing model. I've definitely noticed this with 4.5 and have been putting my Pro subscription to use. I just wish we as an industry cared as much about this side of LLMs as we did with coding and reasoning capabilities.
Even with the right web sources, there's little insight into how AI answer engines use the content of each web source when generating their answers. Similar to the potential for search bias, we lack insight into how each web source is weighed and what other signals are used for determining what to incorporate in responses. Without intentional guidance, LLMs naturally lean toward averaging rather than amplifying unique, minority, or particularly insightful viewpoints.
Okay, maybe we're onto something here. But where does that intentional guidance come from?
Intentional personalization
Tools that grow with you. Tools that understand you.
Today's AI answer engines face fundamental challenges that go beyond the feeling of nostalgia for the old web, surfacing some bespoke notion about serendipitous discovery, or wanting more indie content bubbled up. The real issues—weak attribution, black-box decision-making, and homogenized responses—threaten to flatten rather than enrich how we use the web. Browsing the web isn't and shouldn't be a one size fits all experience.
The current generation of AI tools faces a prodigious challenge: how can they surprise and delight you when they know almost nothing about you?
The solution isn't overwhelming people with more controls, endless configuration opportunities, or exceedingly prominent website cards and attribution. People are confused enough as is selecting the model to use, or having to choose between needing a web search, a pro search, or a deep search, et cetera.
What personalization really means
These tools need to be more personalized to you and tailored to your needs. I know, I know... the term personalization gets tossed around a lot for countless uses—often carrying negative connotations, conjuring images of invasive ad targeting. But there's a better way.
AI personalization doesn't have to be about consuming every detail of your life or cloning yourself. We've fended off giving too much personal data to individual companies, why start now? You don't need to be digitally cloned to help you throughout your day. Even a little bit of info about your past interactions, goals, and interests can go a long way to delivering experiences that resonate more deeply with you.
A better way
This might come as a shock but I don't believe we want to embody some magical "it just works" scenario with personalized AI where these tools know everything about you, build some comprehensive understanding or model of you, and act as you. I believe a more intentional, transparent approach would serve us better, for several critical reasons:
- Behavior ≠ intent: What I do on my computer, phone, or browser is not always what I want to do or who I want to be. That can be a moving target. Just because one user ends up seeing and reading a lot about pop culture news doesn't mean they're not more interested in reading about another topic they're having trouble discovering. This is why it's not as easy as just sucking in as much raw data from the user as possible.
- Context matters: Your needs and motivations can change quickly. They can be based on time, location, task, who you're with, and more. You might be focused on a particular project and don't want personalization to be influenced by what you do at other times. I like to think about this akin to having different profiles in your browser. When researching a specific industry or topic for work, I don't want my personal interests bleeding into my results, and vice versa. This goes much deeper than just a work and home separation.
- Transparency is non-negotiable: Users deserve clarity about how their data shapes their experience. This means showing when personalization is active and providing intuitive controls. Even something as small as highlighting a blurb you enjoyed, requesting more depth on topics of interest, or correcting AI assumptions about your knowledge level.
Providing users transparency and control in tweaking how AI interprets them is critical to seeing, reinforcing, and correcting assumptions as they go. We need to invest in exploring some of these things as an industry. These tools are remarkably complex and we can't hope to nail it on the first try without some light user guidance along the way.
I'm okay doing a bit of work as an end user to shape my experience; and this doesn't just have to be a text response. As a random example, imagine a simplified interest graph representing how the AI understands you, where you prune irrelevant nodes, amplify important ones, and add entirely new focus areas that the system may have missed.
This isn't an entirely new concept, I think we're just so focused on increasingly more capable foundational models and discrete skills like reasoning that we're not quite at the point of going broad enough for things like this. OpenAI has inched in this direction in the past with its Memory and Custom Instructions & Traits features.5
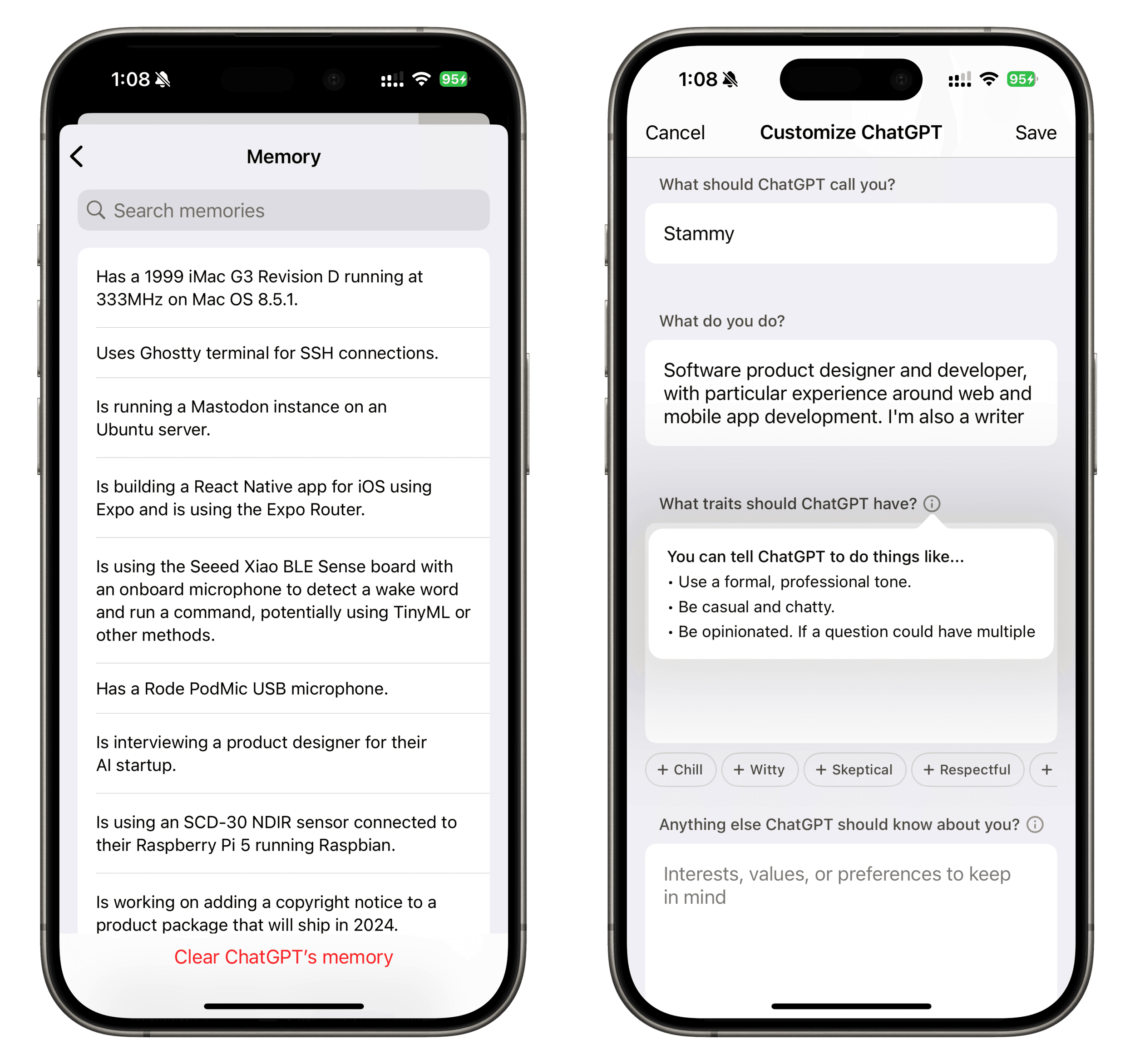
ChatGPT's first steps towards personalization with Memory and Custom Instructions
These current implementations don't yet fulfill the capabilities I've outlined here. For example, it's unclear how ChatGPT decides to save memories, as they often seem to sporadic while also too specific. Additionally, custom instructions are great for high-level information about you, but things like traits feel more task and prompt-specific; not necessarily something I'd always want at the account level.
Personalizing without overreaching
Okay so providing users some control to tweak as they go is all fine... but what kind of source data are we talking about here?
Controlling the OS or the browser are the most powerful positions to be in. While it's hard for most large companies to even think about competing at the OS level, several have begun to compete with browsers: The Browser Company and Perplexity have already announced working on new AI browsers. Maybe OpenAI will enter the arena too.
Even basic browsing data offers powerful personalization potential. What content did you engage with deeply versus merely glance at? What did you create versus consume? Which topics consistently draw your attention over time? These patterns reveal far more than explicit preferences ever could.
There's a lot more to talk about when it comes to data capture here—from OS file access to accessing more detailed info from messaging apps, connecting to various cloud providers (email, docs, bookmarks), or even just knowing what apps someone is using or has installed while accomplishing a task. Some of those can be considerably harder integrations to justify with the additional privacy questions they raise.
I'm already on a bit of a tangent here, but I think the best route, barring inventing your own OS or browser that magically gets massive market adoption, is diving deeper into task-based routes of asking for, clarifying, and showing how context is used (touched on in A better way ↑). It won't be easy though. You'll need to build systems for storing and querying these preferences with each LLM interaction. You won't want to just inject a ton of this as context with every call.
The key is balancing intentional personalization with user agency.
Why does personalization matter again?
Even with light personalization, AI answer engines could meaningfully tailor their responses to you. They would know you're very technical and experienced with the topic at hand to skip the basics and dive deeper into technical concepts. They would know you've been writing about technology for 20 years and really enjoy the underlying ways things work and always want that deeper understanding. And so many other things that might seem like minute details at first, but combined really add up.
They could know enough about you, your past interactions, interests and goals to guide you without overstepping, respecting your time and intelligence. A thoughtfully personalized system would:
- Connect the dots: Help connect new concepts and topics to previous ones you've read about as a frame of reference.
- Identify and manage echo chambers: Help you peek outside the echo chamber by surfacing novel perspectives.
- Leverage trusted sources: Help you learn from web sources you're already familiar with, while balancing new perspectives you may desire.
- Anticipate needs: Proactively suggest content you may be looking for before you've had to ask, in addition to doing tasks for you (The agentic aspect of this type of personalization could warrant an article of its own.)
Technology that knows you, even loosely, and helps you out in these ways isn't a particularly novel concept, but we can't lose sight of it as we design, iterate, and build these AI tools we all use.
Preserving the web's magic
Don't get me wrong, I love these tools. ChatGPT, Perplexity, Gemini, Grok, and others have earned their place in my daily workflow, largely displacing my use of traditional search engines.Yet without thoughtful evolution, they risk homogenizing and muting the web's rich diversity into bland, generic responses—eliminating what made the internet magical to begin with.
The large companies behind these tools have every incentive to develop deeper, more nuanced understandings of their users. In recent months, we've seen firsthand that these companies don't have much of a moat. Switching costs for users are as low as can be: just head to the App Store and pick up the next AI tool topping the charts. We saw it with DeepSeek one week and Grok another.
By adding more intentional personalization to their products, these companies will not only grow their moat and deliver more impactful, tailored answers but also embrace and highlight the web's unique voices and perspectives.
Will it help them directly achieve AGI? Maybe not. But it might start to feel like AGI sooner when these tools are more personalized.
This requires inching towards intentional personalization with refined AI product functionality, greater transparency about how these systems make decisions or apply personalization, and intuitive controls to let users guide their experience without becoming overwhelmed. We can augment human curiosity and creativity rather than replace it.
The magic of browsing the web isn't quite gone, but it's waiting to be reinvented.
1 It's always interesting to see how companies market their AI capabilities. Some really try to downplay AI and make it more about the opportunity, not the technology. Perplexity has more humanizing taglines like "Where knowledge begins", Grok has "Understand the universe", and OpenAI is rather utilitarian with ChatGPT just focusing on uses it can do without a specific tagline (of course OpenAI itself is all about safe and beneficial AGI), and then Google does the opposite and just calls it... "AI mode".
2 I know that is a bit crazy to consider; imagine just visiting a site today and your browser tells you this site is not accurate or has some other editorial issue with the content. That's not the job of a browser or other foundational technology, right? The difference here is that people did not seek this content out themselves, and they don't know what they're looking at or have all the tools available to decide on their own at a glance. The AI answer engine dug that all up on its own, so it's critical to provide as much info as possible to help people be informed about what they're consuming. You can't just put a disclaimer at the bottom saying AI can make mistakes and move on.
3 With every AI company coming out with roughly the same features it's starting to get a bit noisy with all the different modes, let alone models, to use to accomplish your task. That's another problem entirely, but I'm not here to talk about that. I just want to say how amazed I was the first time I used ChatGPT Deep Research. I forked over the $200/mon for the Pro subscription (and I'm still paying for it the next month) and give it a whirl.
It's remarkable how comprehensive it is with just about any prompt. It asks you for feedback before it dives in so you can steer it a bit more which is nice, though the UI for that could be easier than having to reply in a single textarea to a bunch of bullets/questions. ChatGPT Deep Research is the type of thing that gets me really excited about the evolution of these AI tools. They materially save me time and help me go deeper on things that interest me, like learning about topics thoroughly.
4 There was a whole bunch of discourse about this on X, particularly around whether people even want to read stories made by AI. I think we're jumping too far to one end of the spectrum where AI is writing everything. As a writer, I want an LLM that can act as a thought partner. I will provide the ideas, outlines, drafts and I want something to act as an editor, devil's advocate, and mentor all in one. For that role it's key that these tools can actually write well too, not just code, execute python scripts for complex math, and help with reasoning. The way they communicate is critical too. I'm not just thinking about it for writing articles. I want this for helping me frame documents like persuasive product briefs I write, et cetera.
5 Another sign that OpenAI is thinking about personalization was spotted in their recent announcement for the new Responses API. They showed a brief example use case for the file_search
tool to gather user preferences for an agentic task. The exact implementation was left up to the API consumer in that example of course. While this article is generally about AI answer engines and the web, personalization like this will be especially important for agents.
The title of this article is a reference to an immersive theater work called Sleep No More I saw in NYC a few years ago. It tells the story of Macbeth and there's some interesting parallels I'm drawing on from this article. For one, loss of agency. When you visit you're immersed in an environment where you feel like you lose control (similar to what AI answer engines feel like they're doing today). You're a spectator wandering around while confusing things happen around you.